2 min read
Taking on the future: Machine Learning in FX Risk Management
Millennium Global Mar 28, 2024 1:36:32 PM
By Justin Gang Xu, Head of Risk
The global foreign exchange markets are primarily influenced by a combination of fundamental and market dynamic factors. Fundamental factors may encompass economic drivers like inflation, interest rates and terms of trade, while market dynamics could consist of factors such as market sentiment, consensus positions and technical indicators. When developing currency risk management strategies, one well-established approach is to generate trading signals based on the above factors and weight them accordingly to ensure the strategy can efficiently capture the market drivers while remaining well diversified and adaptive to the changing market conditions.
AI: a helping hand or added risk?
Recent advances in Artificial Intelligence (AI) have provided market participants with enhanced capabilities to identify FX market drivers through the adoption of alternative data sources. For example, market participants traditionally relied on metrics such as volatility, options skew, or market surveys to assess currency market sentiment. With the introduction of new technologies such as Retrieval Augmented Generation (RAG), it has now become possible to accurately gather information from a much wider range of financial documents, reports, and news articles through large language models supported by RAG and prompt engineering. Using technologies such as FinBert (Bert transformer architecture fine-tuned using financial corpus) enables market participants to gain a deeper understanding of market sentiments, such as changing of central bank rhetoric on inflation, growth and labour market dynamics.
In addition to identifying market drivers, machine learning algorithms can also be utilised to enhance the investment decision-making process by exploiting the nonlinear interactions of market drivers. Tree-based approaches (either parallel trees or boosting trees) can handle a large number of market drivers, and are particularly adept at capturing non-linear relationships between the drivers and exchange rates.
One important factor to consider when developing machine learning algorithms is data snooping. It is vital to ensure the input variables are well motivated and go through a rigorous feature selection process. In addition, robust governance should also be established around model development, deployment, evaluation and improvements that not only considers model performance, but also model transparency, explainability and interpretability.
Artificial intelligence and machine learning enable market participants to identify price patterns and correlations via analysing vast datasets. It allows the inclusion of alternative datasets such as texts, documents, images and videos etc. By integrating AI and ML into existing processes, it offers significant potential to broaden the market drivers and enhance FX risk management decision-making capabilities of businesses.
Millennium Global Investments has a long track record of developing quantitative FX absolute return and risk management strategies. We are actively exploring using machine learning and artificial intelligence techniques to broaden access to different data sources and enhance our investment decision making process to achieve superior risk-adjusted returns for our clients, while maintaining a rigorous focus on risk management and risk controls.
The document contains the views and opinions of the author for discussion purposes only and does not represent that any of the products/strategies managed by Millennium engage in AI. This information is intended for Professional Clients only, not retail.
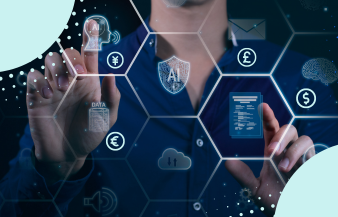
Taking on the future: Machine Learning in FX Risk Management
By Justin Gang Xu, Head of Risk The global foreign exchange markets are primarily influenced by a combination of fundamental and market dynamic...
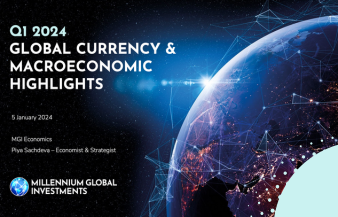